Logpodge posts are round-ups of ideas that donβt work as a full essay and that I couldnβt squeeze into Notes.
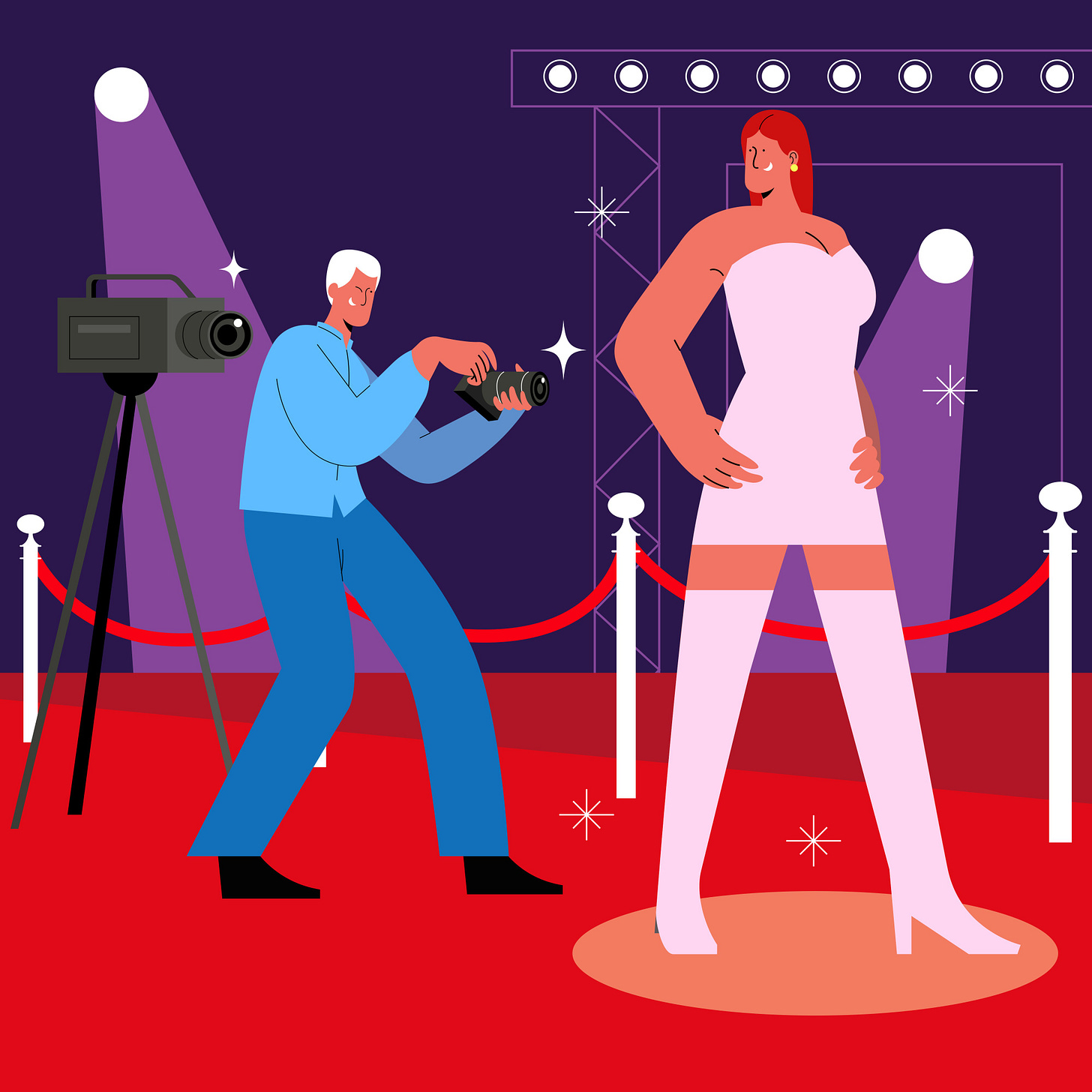
Disclosures: I talk about my work as a consultant in this piece, so I need to tell you about my disclosures page and this essay explaining how I make a living while writing on the internet.
The boring AI revolution
Iβve decided to follow these folks and use the term βlarge AI modelsβ instead of LLMs and the alphabet soup approach to describe ChatGPT, Claude, and the like. Brad DeLong calls them MAMLMsβModern Advanced Machine-Learning Models in his easy-to-miss-but-well-worth-finding commentary on what these models can and cannot do. His recent take that βmuch smaller LLMs than we already have are more than sufficient as natural-language front-ends to structured and unstructured dataβ rings true.
This is what I see in my consulting work. LLM-based solutions need not use the latest and largest models for many problems that need solving. Leepfrog Technologies was doing machine learning when it was still called machine learning. Iβve been working with them as they developed and deployed a new feature in their curriculum management software, which allows a faculty member proposing a new course or an academic administrator reviewing a course proposal to search for similar courses using an LLM-based tool. Unless I shout βAI optimizationβ or call it βa revolution in curriculum developmentβ that sounds boring. But it is not for someone who wants to gauge what similar courses are in development or already offered on campus.
LLMs are not so good at doing the work of human tutors, but they are good at using word vectors to present relationships among combinations of words. If you constrain the results to a limited, structured database with links, confabulations are not a problem. The problem this solves is that keyword searches of titles and course descriptions do a poor job of connecting similar ideas across disciplines and rely too much on specific words and combinations of words. Also, youβll be amazed to hear that impenetrable jargon sometimes appears in academic course listings. This tool does not exactly solve that problem, but a jargon-to-plain-language translator would make a great feature for the interface to a course catalog.
This may sound boring but it is also a real thing that actually existing AI technology can do. In fact, it is something large (but not the largest) AI models can do, including models that are open source, run locally, and not expensive.
Explorance is another company that was doing machine learning back when it was called machine learning. Iβll be giving a talk titledΒ The Boring Revolution: How AI Will Change Education and the Workplace, and Why You Might Not Even Notice at Explorance World 2025 in June. Youβll see writing on this theme here on AI Log as I try to figure out what to say. Right now, my plan is to walk around the stage waving The Unaccountability Machine while talking about the management singularity.
Boring AI journalism
Journalists do not care to write about the boring uses of large AI models or companies that make effective but boring use of large AI models. If it is not scary in the way of AI is coming for your job or AI will make you its sex slave, then itβs not news. Occasionally, though, the boring stuff sneaks into an article because the headline sounds scary. Like this one from The Markup:
For the First Time, Artificial Intelligence is Being Used at a Nuclear Power Plant: Californiaβs Diablo Canyon
That sounds frightening until you read the article and find out that
the artificial intelligence tool named Neutron Enterprise is just meant to help workers at the plant navigate extensive technical reports and regulations β millions of pages of intricate documents from the Nuclear Regulatory Commission that go back decades β while they operate and maintain the facility.
I donβt blame The Markup for this. They needed a headline, and βfirst time + AI + Nuclear Power Plantβ is attention-grabbing. The reporting is not alarmist. It explains that this is a boring but useful solution to a class of problems that any organization operating in a highly regulated sector of the economy faces: millions of pages of intricate documents going back decades. Word vectors and natural language processing greatly improve your search toolβs chance of finding what youβre looking for. I have not seen any numbers on this sort of thing because there are few incentives to ask such questions, but Iβd bet all my money on Manifold Markets that this use of LLMs is saving more employee time than Microsoft Copilot.
Higher education is also a highly regulated industry. In my former days as a bureaucrat, much of my time was spent arguing with people about what was and what was not allowed by rules and regulations. Often, the argument revolved around some other bureaucratβs half-understood version of what a lawyer told him. You had better believe that putting your actual eyeballs on the relevant regulation or accreditation standard was useful. I spent a lot of time searching government and accreditation websites for the right document.
The AI enthusiast line that what we use today is the worst AI we will ever see misses that. for many problems, all we really need is AI from two years ago.
Large AI models have large elbows
OpenAI and Anthropic have enlivened the already lively AI as edtech market by deciding to sell directly to institutions of higher education. I wrote last summer about ChatGPT Edu using the gold rush cliche, saying
OpenAI could have let everyone else figure out how to make products that schools would buy and simply avoided the hassle of negotiating privacy guarantees and the tricky work of price discovery. They could focus on renting the picks and shovels in the form of access to the best foundation model and let others pan the streams and mine the education hills.
Instead, OpenAI decided that direct access to students is valuable enough to spin up its own mining operation.
Anthropicβs Claude for Education and OpenAIβs announcement of free sign-ups for ChatGPT Plus are indications that both are throwing their large elbows at startups who put Socrates masks on large AI models and sell them as the future of teaching. Why wouldnβt Anthropic just do that and call it learning mode?
Institutions that decide to pay for this service are making a bet that chat interfaces hooked up to a large AI model will offer some value as an educational technology. Given how little we know about how students are using AI, it seems weird to pay to get everyone a subscription to βlevel the playing field.β What if it turns out that access to large AI models is correlated with poor academic performance?
There are a lot of reasons why signing on with OpenAI or Anthropic is a bad idea, but the obvious one is that the market has changed. In case you didnβt notice, itβs bad for colleges and unversities. Institutions have more pressing issues than figuring out AI. I wonder how much this came up at ASU+GSV Summit 2025. Itβs hard to think about anything when youβre busy learning at the speed of light. While everyone else thinks about the likelihood of a recession, higher education is already cutting back. Who exactly is going to buy an institutional license for an experimental product during the lean times?
I havenβt seen any reporting on the financial aspects of Anthropicβs partnership, but it looks to me like an answer to OpenAIβs NextGenAI, a case of AI giving money to universities to experiment with their products. This strikes me as an excellent way to spend investorsβ money, though Iβm not sure it bodes well for AIβs profitability.
If I had both companies offering me goodies, Iβd choose Anthropic. They get credit for being more open about their research and for not being run by Sam Altman. The education report Anthropic released yesterday is no game-changer. It fits my prior assumptions that computer science and students in STEM are where most of the student action is and that when you get rigorous about it, you find that the actual number of people using large AI models for education is relatively small. Credit Anthropic for actually talking about cheating and for saying their analysis βsuggests that educational approaches to AI integration would likely benefit from being discipline-specific.β What! No? You really think so?
It is never too late to learn what your customers actually do. Well, thatβs not true, as weβll see when the AI edtech companies discover what happens when the pools of capital they have been swimming in dry up.
AI Log, LLC. Β©2025 All rights reserved.