Note: This follows On Beyond AGI! where I explore the idea that large AI models are cultural and social technologies as a much-needed alternative to endless debates about artificial general intelligence.
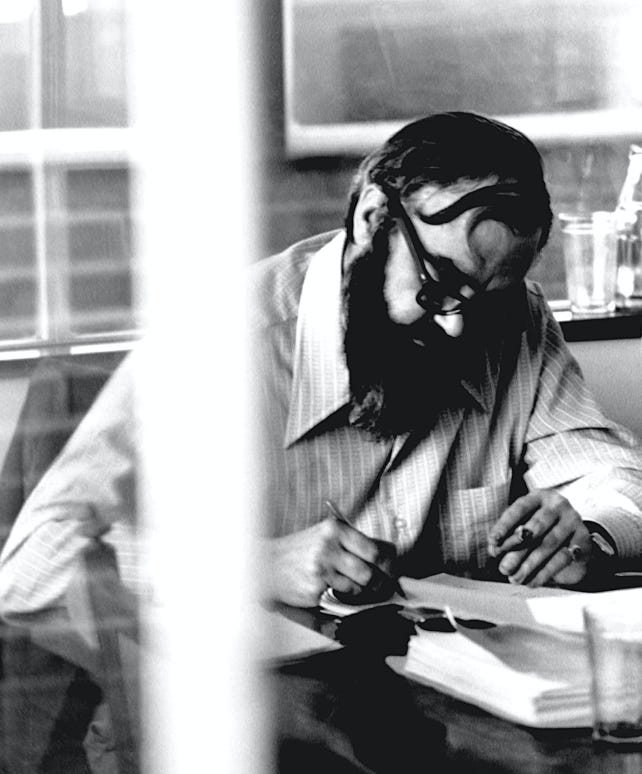
The ignoring of data is, in fact, the easiest and most popular mode of obtaining unity in one's thought. —William James
Is Management Cybernetics becoming the new hotness?
Scott Alexander spends 800 words pretending he doesn’t understand the rhetorical function of an aphorism. The one-liner in question Stafford Beer’s “The purpose of a system is what it does” (POSIWID). Instead of thinking about Beer’s ideas or exploring the early days of a revival of management cybernetics, Alexander takes the easiest possible path for someone writing on the internet for fun and money: he dunks on random Twitter users.
Nice work if you can get it, as the song goes.
Kevin Munger of
takes the time to explain the slogan’s historical context and rhetorical purpose to Alexander’s audience, but the news here is that Alexander saw value in badmouthing a guy who died in 2002 because people were talking about him. As Munger says, “I'm glad that Stafford Beer is getting famous enough to be vacuously quoted by random people on Twitter!” If one of the more successful bloggers of the past decade and a bunch of shitposters decide Beer is worth their attention, then maybe something is happening here.Though Alexander doesn’t mention it, one reason Beer’s mix of poetry and math is in the digital air is The Unacccountibility Machine, Dan Davies’s hilarious application of Beer’s ideas to our current social problems. The University of Chicago Press just published a US edition of the book for the low, low price of $20. Among the amusing stories and insights about the making of our current crisis, Davies argues that Beer can help us think beyond the fading Freidmanite belief that satisfying shareholders’ desire for profits is the sole purpose of a corporate entity. Replacing this narrow aim with something more expansive than the kleptocratic enrichment of the already rich requires new and different ideas along with methods to realize those ideas.
Management cybernetics, the application of information theory to organizational change management, helps think about the tension between the stated purpose of social institutions like corporations and universities and the outcomes they produce. For those interested in rebuilding whatever is left of civil society four years from now into something that aims for the public good, revitalizing Beer’s ideas and methods affords opportunities for turning thoughts into action. I sketched this in the context of higher education in this review essay of The Unaccountability Machine and
’s Failing Our Future.Mobilizing to do this work after ChatGPT requires updating our slogans. As Munger says, POSWID has pretty much exhausted its power “thanks to decades of public choice economics and the general demystification/disenchantment of our institutions.” Perhaps, large AI models are cultural and social technologies (LAIMACAST) is the new POSWID. Maybe we dispense with acronyms: Make unaccountability machines accountable to the people! Or, we could add an exclamation point to Arvind Narayanan and Sayash Kapoor’s new slogan: AI is normal technology!
Whatever we land on, it should replace the endless arguing over artificial general intelligence as the vague and entirely speculative explanation for what’s going to happen next. AGI has the three-letter advantage, which, as any student of acronyms knows, hits the TLA sweet spot. As more writers like Josh Brake and Gill Kernick engage with The Unacccountibility Machine, we will find ways to abbreviate management cybernetics for a wider audience without sacrificing too much of the complexity at the heart of the enterprise.
Escaping AGI through history
Instead of treating AGI as something up for debate or assuming it (whatever it is) will happen one day, we should collectively giggle at the idea that a machine that applies probabilistic mathematics to giant cultural datasets can only be explained using the terminology of human cognition. As Cosma Shalizi pointed out, attention, as it is used in that famous paper, does not mean what you think it means. “Calling this "attention" [is] at best a joke. Actual human attention is selective.”1
Brad DeLong highlights this wonderful collection of insights about attention on his way to exposing the lazy thinking that anthropomorphizing large AI models affords otherwise smart people. DeLong offers his own “more accurate and useful framework,” which is “to understand LLMs as flexible interpolative functions from prompts to continuations.” Thinking in those terms requires greater cognitive effort than using the tired analogy of AI models as confabulation-prone research assistants or weird interns, but it has the benefit of agreeing with the reality of what we know about how large AI models actually work.
With every chatbot interaction, with every picture, song, or video generated, the evidence for understanding large AI models as social and cultural tools gets harder to ignore. Shalizi and DeLong are contributing to what Henry Farrell calls “a shared terrain of ideas coming into view,” which places generative AI within history rather than as the leading edge of an epoch-making inflection point that changes everything.
As satisfying as it is to yell “you’re full of shit” at the true believers and con artists preaching AGI, our efforts are better spent thinking about large AI models as “a normal technology” being used by what Ada Palmer calls an “information revolution species.” Instead of talking about the singularity, we should think in terms of Alison Gopnik’s parable of AI as collective intelligence accessed through an information tool built out of “gradient descent, next-token prediction, and transformers.” This specific technology is new, but humans have been inventing cultural tools since our ancestors decided to paint images on cave walls.
As Palmer says, comparing what’s happening now to the printing press:
This revolution will be faster, but we have something the Gutenberg generations lacked: we understand social safety nets. We know we need them, how to make them. We have centuries of examples of how to handle information revolutions well or badly…The only sure road to real life dystopia is if we convince ourselves dystopia is unavoidable, and fail to try for something better.
Using a framework that treats generative AI “as analogous to such past technologies as writing, print, markets, bureaucracies, and representative democracies” means revising how we approach the creation and extension of social safety nets while avoiding dystopia. As DeLong has argued clearly and at length, we figured out 150 years ago what looked like the hard part: how to create the material conditions for everyone on Earth to live well. For political, social, and cultural reasons, we don’t seem able to meet the challenge of the next step: redistributing material wealth such that everyone gets a reasonable share. This emerging terrain of ideas about managing large social institutions using large AI models offers us the opportunity to evaluate how large AI models might address the constellation of public problems we humans face.
Superhuman intelligence will not arrive in the next generation of large models and is highly unlikely to arrive any time soon, so it will not help us face the crisis now occurring. Yet, this crisis will produce real change. We need ideas to guide that change. As Corey Doctorow says, “Milton Friedman was wrong, but he wasn’t wrong about this.” Large AI models as a social and cultural technology and cybernetics management are among the best ideas lying around. Let’s take them up and see what change we can make.
AI Log, LLC. ©2025 All rights reserved.
Since I am in the habit of dropping William James into discussions of AI, I want you to know that the link to Principles of Psychology is original to Shalizi’s essay.